Facial Recognition: Surveillance with “Artificial” Intelligence
Hi-tech is often supported by usually invisible, manual, and exhausted workers whose work practice implies something far from hi-tech.
Written by Jack Linzhou Xing
Published on 23/01/2021
“Want to buy a house? Better wear a helmet when you go to the sales office!”
Such a famous saying in the recent Chinese Internet space, seemingly weird and even absurd, originated from a piece of news about facial recognition. According to multiple official medias such as South China Metropolis Daily, some real estate sales office adopted the surveillance technology of facial recognition, which could figure out whether a certain client came “voluntarily” or via the introduction of sales agents, so that they could adopt different pricing strategies to different clients. It was reported that “voluntary” clients could suffer from a price higher than normal by hundreds and thousands of RMB.[1] This news, exemplifying many other reports about facial recognition, raised great concerns about privacy especially in the Chinese society famous for civil and governmental surveillance systems.
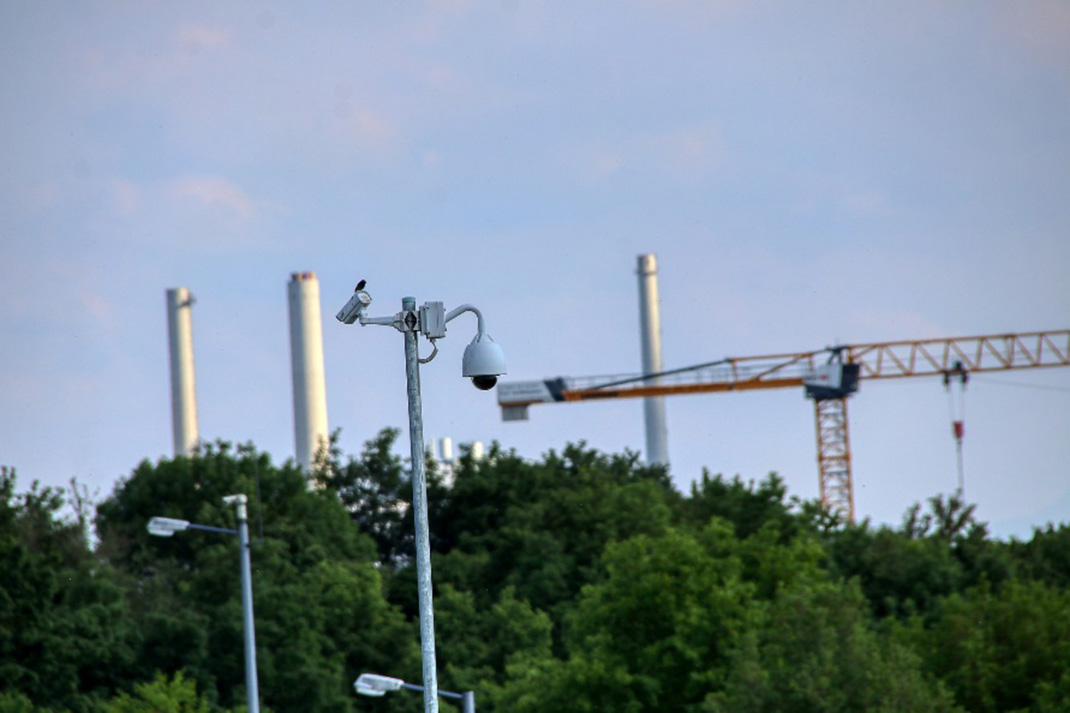
The basic principle behind facial recognition is about extracting facial characteristics from images of a certain person’s face, and judging the similarity of this face to the data of faces stored in a database. In recent years, due to the rapid development of machine learning, the capability of facial recognition algorithms and devices has significantly developed.
The standards of good facial recognition algorithms and devices are mainly twofold. The first one is the speed of smoothness, while the second one is accuracy. In the following parts, we will discuss the implications of the two standards one by one.
Smoothness: Disappearing Consent in the Usage of Facial Recognition
Smoothness requires facial recognition devices to be fast and traceless, just as what happens in interpersonal interactions. When interacting with somebody by our eyes, we simply look at the person and “intuitively” recognize him or her. Similarly, when you pick up your phone, it should unlock itself as fast and traceless as someone recognizes you.
Nevertheless, devices are not people. Such an interaction, if happening between machine and people, may risk becoming “non-interaction”. That is to say, when the device recognizes us, it does not get our consent. This situation of non-interaction is rare even in the current society where people’s privacy has already been seriously threatened by many digital technologies: When passing the luggage screening, you are subject to the surveillance of the screening machine, but you at least showed your consent by putting your luggage into the machine. When browsing YouTube, you are subject to the recommendation algorithm, but you at least showed your consent by the action of browsing the videos you like. Whereas when you walk on the street randomly, you do not necessarily know that you might be in the scope of a facial recognition camera. Therefore, whether you want it or not, whether you consent or not, your information is caught by the facial recognition device when you simply appear before it.
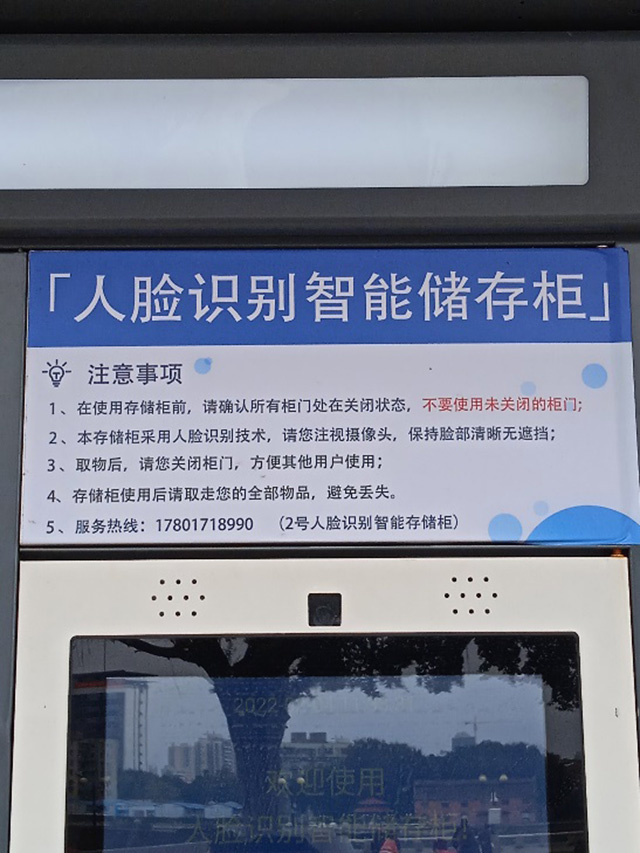
Therefore, the smoothness of facial recognition deserves even more concern about privacy. When information can be collected virtually with no consent, the possibility of leakage and misuse increases significantly. After all, we may have “lost” our facial by simply walking down the street.
Accuracy: Artificial Intelligence or “People-made” Intelligence?
The accuracy of facial recognition algorithms and devices does not come from the vacuum. After all, artificial intelligence does not naturally equal intelligence. In Chinese, artificial intelligence can be literally translated into “rengong zhineng (人工智能),” in which rengong means “created or maintained by people.”
So, who created the intelligence? The answer is hundreds and thousands of data annotators.
The accuracy of facial recognition depends mainly on two factors. First, the competence of the algorithm. Second, the size of the database. In the era of machine learning, algorithms are trained with a mass amount of data to get their competence improved. Meanwhile, the larger the size of the database, the more precise can the algorithm match the image in the camera with a face in the database. Therefore, both factors require a mass amount of data of face annotated with “key facial data points.”
China, typically having relatively loose privacy policies, a mass amount of surveillance cameras, and a large supply of mid-to-low skilled workers, becomes a hotbed for the data annotation industry. Currently, there are altogether 20 million surveillance cameras according to the country, each producing GBs of facial data per day.
These data are transferred to data annotation companies which organize hundreds or thousands of workers to sit in front of computers, check one picture and another, and mark all the key facial data points of each picture. According to Jiazi Light-Year, a tech-focused think tank, there are currently 100,000 full-time data annotators and even more part-time ones in China, with a rapid speed of growth.[2] One of the most famous data annotation companies, BasicFinder, has established data annotation “factories” in Beijing, Henan, Hebei, Shandong, and Shanxi, and recruited over 2,000 workers. Besides, they also outsource part of their orders to other companies in smaller sizes or even small informal data annotation teams.
The data annotation work, just as any “low-end” jobs typically taken by migrant workers, are characterized by low pay and exhausting work practice. According to the investigation by Initium Media, a typical worker in the “factory” in Beijing can make around RMB 4,000 to 5,000 per year.[3] Given the fact that many of the workers are rural migrants and have rural dependents, their actual personal disposable income is much lower than the average disposable income per capita of urban Beijing, which was RMB 6,154 in 2019.[4] In the case of small companies and teams depending on outsourced orders, workers’ income can be even lower given the transaction costs generated in the process of outsourcing.
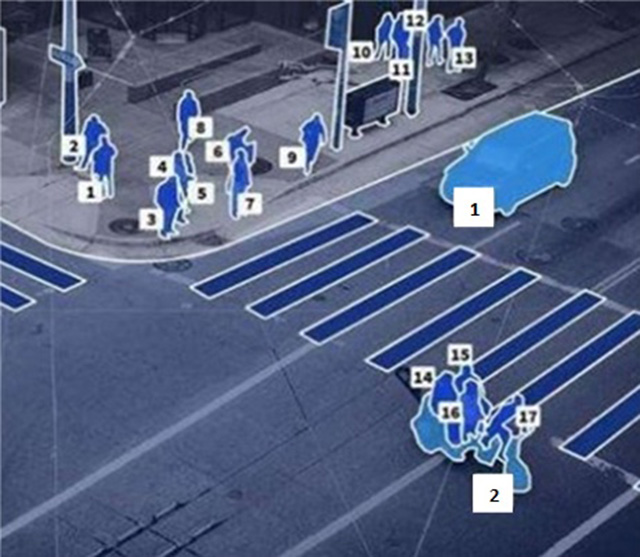
With such low income, workers need to sit in front of the computers for ten hours per day, stare at the screen with a high degree of concentration, mark or circle out detailed data points on pictures with usually very low dpi. More or less surprisingly, the majority of workers do not understand why certain data points on a facial picture are key for the algorithm to judge the identity of a person. For them, what points to mark or circle is only a matter of top-down instructions, and they usually do not care about the reason and the scientific principles behind the selection of data points. The highly manual and physically exhausting work practice forms an ironic and sharp contrast to the hi-tech nature always claimed by companies that sell facial recognition algorithms and devices.
Despite the low pay and exhausting nature, the job of data annotation is still ideal in many young workers’ eyes. According to Initium Media, workers in the data annotation factories treasure the job because it is at least a formal and stable job in an industry with a bright future. Moreover, the nature of the job, which involves the usage of computers and a high level of patience and concentration, makes the job look high-end and not as easy as the outsiders think. This brings some occupational pride to the workers. The third reason lies in the significance of the job. Workers are told – and it is true – that the quality of their annotation is highly related to issues including whether the algorithm can accurately figure out thieves in public sites and sense the dangerous traffic condition. This provides an additional sense of pride and responsibility to the workers.
Similar to the workers, local governments in relatively backward areas of China, such as Guizhou Province, see the data annotation industry as a great opportunity for the development of the local economy. The fact that the “product” of data annotation can be transferred in no time via the cloud effectively mitigated the traditionally deadly disadvantage of inland, remote, and mountainous regions (Science and Technology Daily 2019).[5] With normal buildings, hundreds of computer devices, and smooth Internet connections, local governments in Guizhou are glad to attract Guizhou migrant workers back to their hometowns.
No matter whether the facial recognition technology is intelligent or not, at least, it is literally “artificial,” i.e., made by the hard work of many people.
Takeaways: Social Infrastructure for the Loop of Facial Recognition
The two standards of facial recognition, smoothness and accuracy, coincidentally indicate a hidden loop about how the facial recognition technology can operate and develop, especially in societies like China. To achieve smoothness and accuracy, the technology requires a loose legal and policy environment regarding regulations of privacy, so as to get a mass amount of data supply. It also requires a large supply of mid-to-low skilled workers who can bear the exhausting work and relatively low income. When it is widely applied to society, it further raises concerns about the potential degradation of people’s privacy.
It is not surprising that China can lead the world in facial recognition. After all, it meets the abovementioned two requirements, and provides a wonderful social infrastructure for the operation and development of facial recognition.
What should we do? At least, we need to always remain cautious to the potential impact of hi-tech to our privacy, and always bear in mind that hi-tech is often supported by usually invisible, manual, and exhausted workers whose work practice implies something far from hi-tech.
Footnote
- South China Metropolis Daily. 2020. “Jiemi shoulouchu renlian shibie ‘shashu’ (Revealing how sales offices use facial recognition to price real estates to the disadvantage of existing customers).” Accessed Jan 23, 2021, from: https://m.mp.oeeee.com/a/BAAFRD000020201123383330.html.
- Jiazi Light-Year. 2019. “Cong xiao zuofang dao da shengchan, shuju biaozhu zhuanlidian (From small workshops to mass production, the turning point of data annotation).” Accessed Jan 23, 2021, from: https://zhuanlan.zhihu.com/p/97613019.
- Wu, Jing. 2018. “Shuju gongchang li de biaojiyuan: women xunlian rengong zhineng, zhidao tamen qudai women (Annotators in data factories: We train artificial intelligence until they replace us).” Initium Media. Accessed Jan 23, 2021, from: https://theinitium.com/article/20180522-mainland-data-annotator/.
- Beijing Statistics Bureau. 2020. “Jumin renjun ke zhipei shouru qingkuang (Situation of disposable income per capita for residents).” Accessed Jan 23, 2021, from: http://tjj.beijing.gov.cn/tjsj_31433/yjdsj_31440/jmsz_32036/2019/202002/t202002 17_1647244.html.
- Science and Technology Daily, 2019.